Introduction To Production Snapshots
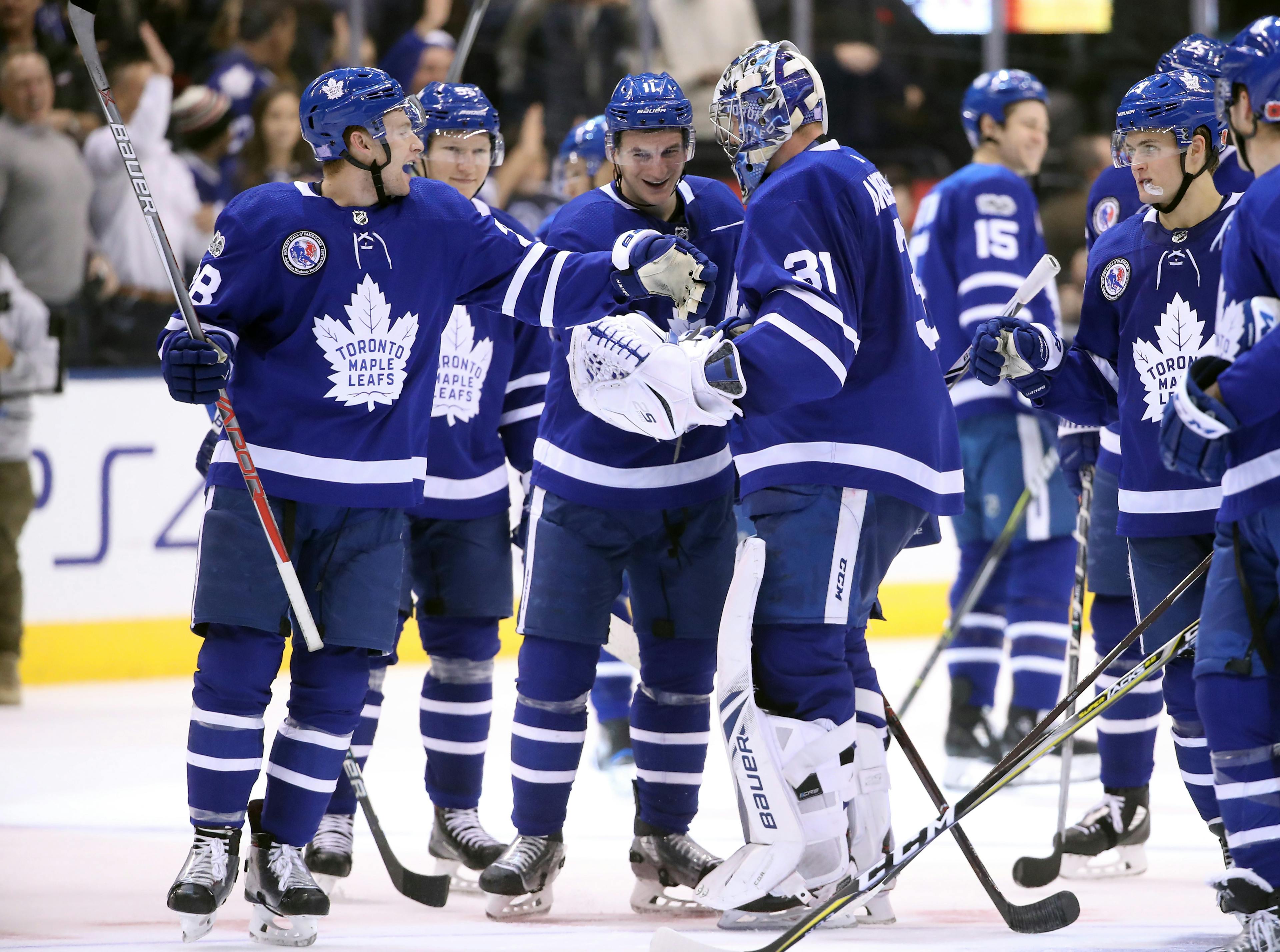
By Ziggy
6 years agoEvery once in a while, someone on twitter asks me how to interpret my Production Snapshots. I usually try to explain in a tweet or two, but now seems like a good time to make a full explainer. In this post, I will walk you through the main aspects of a Production Snapshot, starting with a general overview of how the sample size is constructed and how you can use them, and then I will narrow our focus down to the meanings of the particular numbers. By the end of this post, you will be able to interpret Production Snapshots on your own, and perhaps you will be introduced to a new stat or two along the way.
The ultimate purpose of a Production Snapshot is to provide you with a quick and easy summary of how a particular forward produced in a given period of time. Personally, one of my goals with these snapshots is to convey hockey stats in a way that you can understand, regardless of your familiarity with the stats themselves.
The Sample Size:
Each stat in a NHL Production Snapshot has a sample size of 403 forwards. Although this number may seem random to some of you, finding it was actually quite simple. We know that a NHL team has 12 spots in their lineup for forwards (4 lines with 3 forwards on each line). For our purposes, we also want to leave room for one extra forward per team. (The healthy scratch, if you will). This leaves us with 13 forwards per team. And in a league with 31 teams, we arrive at the number of regular forwards in the league: 13 x 31 = 403. To filter out the forwards who don’t play very often, I selected the 403 forwards with the most ice time at 5-on-5 this season.
While this is a common way of finding the sample size in hockey analysis, it also gives us a nice way to interpret the data. Our sample size reflects the size of a typical NHL lineup, so we can actually break it down into 5 subgroups: 1st line, 2nd line, 3rd line, 4th line, and the 13th forward.
Using the same method I used to find the entire sample size, we can quickly find the size of each subgroup. Since a typical NHL team has three forwards on the first line, and there are 31 teams in the league, there are 93 first line forwards in the league. In our sample of 403 forwards, this subgroup of 93 forwards composes roughly 23% of the entire sample size. We can treat the best 93 results in a particular as “first line caliber” results. The corresponding percentiles for first line caliber results range from 100 (1st overall) to 76.92 (93rd overall). (Note: this is what you see in the bottom-left box of NHL Production Snapshots).
From here, it is easy to see how the other lines are constructed. After the best 93 results, the next best 93 are “2nd line caliber” results (i.e. results between 94th overall to 186th overall). The 3rd and 4th lines must obviously be the same size, so they both contain 93 results each. Results that are “4th line caliber” or better compose 92.3% of the sample.
The other 7.7% belongs to the subgroup containing the worst 31 results, the “13th forward”. This subgroup exists because we included 13 forwards on each team when we created our sample, rather than 12. The following table is a breakdown of the sample size into its 5 subgroups:

With these 5 subgroups in mind, we can see how to interpret the percentiles in a particular Production Snapshot. The cells in the “Percentile” column contain the percentiles of a players results in every stat. In the snapshot below, for example, we can see that Auston Matthews’ scoring rate is better than 98.76% of the entire sample.
In fact, Auston Matthews’ results in every stat included in the chart are first line caliber. We can see this at a glance because the cells in the “Percentile” column are coloured dark blue, in accordance with the colour scheme in the bottom row. The only exception is the percentile for 5v5 ice time per game, where the background is always gray and the colour of the text itself changes. I did this because a player cannot control his ice time, but it is still useful to compare his ice time to his production in those minutes. For this reason, the text itself follows the colour scheme instead of the background.
While Auston Matthews is clearly producing like a first line forward, Production Snapshots are also good at detecting unproductive players too. A player whose results are mostly in the final subgroup (i.e. 13th forward, coloured red) is one of the least productive forwards in the league; Leo Komarov is currently a good example of this. In this way, Production Snapshots illustrate where a players results would rank on a typical NHL team.
Now that you understand the underlying features of Production Snapshots, there are a few important things to keep in mind before you use them.
Warnings:
1. The power of this format creates opportunities to make sweeping conclusions about a player by quickly glancing at a chart. Please don’t do this. One chart does not have the power to completely illustrate a players skill, and I’d be lying if I claimed otherwise. Of course, the snapshots are a quick and fun way to analyze players, but they are meant as a starting point for your analysis. If you are not also watching the games or thinking for yourself, you are doing it wrong.
2. Important information about each chart can be found in the top-left cell. This information usually pertains to which stats are adjusted, the dates associated with the stats, and the source of the picture in the background.
3. I should also note that the sample is not necessarily the same 403 forwards for every stat. The number of samples depends on the number of places the data is from. In these particular snapshots (i.e. the NHL ones that include data up to and including games on December 31, 2017), all the data is from corsica.hockey, so there is only one sample. For versions of these charts that use multiple samples, however, you will sometimes see players with stats missing, like here. This happens because they are in one sample but not the others.
Stats Glossary:
Of course, it is impossible to learn anything from Production Snapshots without understanding the stats themselves. Here are some of the most common stats you might find in a Production Snapshot:
Scoring Rate (G60): The number of goals scored by the player for every hour of ice time he plays.
Primary Assist Rate (A160): The number of primary assists awarded to the player for every hour of ice time he plays.
Primary Points Rate (P160): Scoring Rate + Primary Assist Rate
Individual Shots (iCF60): The number of shots (including blocks and misses) taken by a particular player every hour of ice time he plays.
Expected Scoring Rate (ixGF60): How often you would expect this player to score at 5v5 in the long-run. This expectation is largely based on the locations of his shots.
Game Score per hour (GS60): A metric designed to measure single-game productivity. You can read more about it here. Like all other stats in the chart, it is expressed as a rate stat.
5v5 Ice Time per Game (TOI/GP): The average amount of 5v5 playing time this player receives each game.
If you have any questions, feedback, or chart requests, leave a comment below or feel free to reach out to me on twitter through a tweet or a direct message.
Recent articles from Ziggy