Using Adjusted PPG to Evaluate the 2017 NHL Draft Class
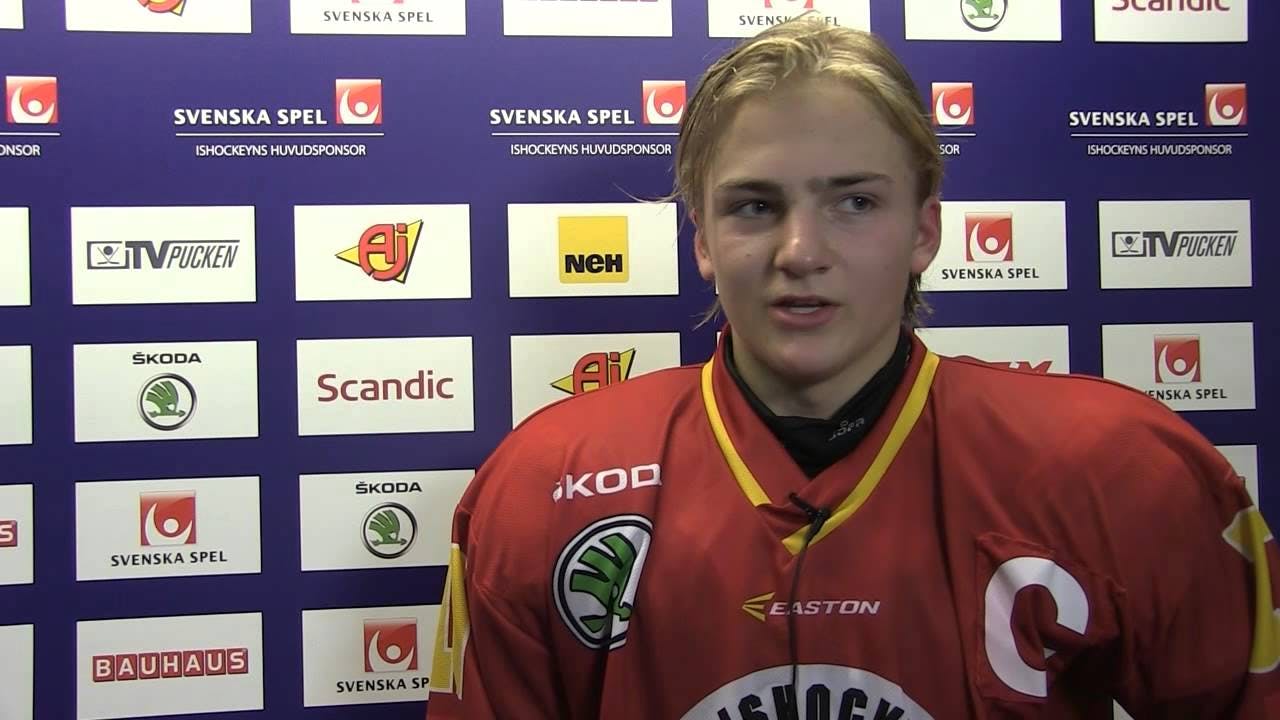
By Ian Tulloch
6 years agoLast week I wrote about the revisions I’d made to NHLe, which I think help provide a more accurate reflection of European leagues’ strength relative to each other. Arguably, the most difficult aspect of evaluating draft-eligible prospects is comparing players in North American junior leagues (ie. the OHL) to players in European Leagues (ie. the SuperElit). It’s so tough that NHL Central Scouting splits their rankings into two lists: North American skaters and European skaters (they also have separate the lists for goalies, but we won’t be engaging with voodoo today).
With the new NHLe translation factors from last week’s piece, though, we can make semi-accurate adjustments for league strength, allowing us to compare point production between players in North American and European leagues. Keep in mind that when we look at scoring rates, context is extremely important (TOI/PP usage/QoT/QoC will significantly impact prospects’ production), so we’re definitely not looking at an all-encompassing measure. Think of it more as a way to get a rough estimate of how impressive a player’s point production is based on their league difficulty.
The Methodology
As a quick reminder, here are the updated NHLe translation factors for European leagues using the Wilson Method.
And here’s a list of the translation factors for North American leagues based on Rob Vollman’s latest NHLe update:
Since the OHL is probably the most well-known junior league (and because of my #TorontoBias), we’re going to use it as our baseline for point production. What this means is that we’re going to take every prospect’s point production (Points Per Game), multiply it by the NHLe translation factor for their league (ie. 0.25 in the SuperElit), and divide it by the translation factor for the OHL (0.31). This is going to serve as our league adjustment.
In addition, I felt it was important to make an age adjustment. Before selling his soul to become a Computer Boy™, Rhys Jessop did some excellent work on the impact of age on draft eligible prospects’ point production. He found that even a few months can make a huge difference. For example, a player who is 17.9 on September 15th of their draft year is much more physically developed than one who is 17.1, giving the older player an unfair advantage. To account for players at different stages in their development, we’re going to use Jessop’s age adjustment. For the mathematically inclined, here’s his equation:
Age-Adjusted Pts/GP = (1 – ([Age as of September of draft year – 17] * 0.1672)) * Pts/GP
The Results
So without further ado, here’s the list of players’ League & Age Adjusted PPG (note that this list is only for players in their draft year – I’ll be putting out the list for overagers next week). For transparency, here’s the Excel file I used for the calculations. I also included a column in the table showing where the players ranked in Future Considerations’ final Top 100 rankings. I thought this would help provide some context in regards to how well these prospects are regarded by scouts.
For fun, let’s dive a bit deeper into these numbers. I don’t want to put too much data in one image, so we’ll look at the Top 30 in Adjusted PPG. Let’s start with the forwards.
Forwards

This list does a great job highlighting some of my favourite high scoring prospects that I believe are undervalued in most public draft rankings (ie. Suzuki, Pettersson, Yamamoto, and Robertson). It’s also interesting seeing some players that draft nerds like myself are much lower on than the scouting community (Mittlestadt, Rassmussen, and Tolvanen come to mind).
This isn’t to say that stats are always right and the eye test is always wrong; both are integral components of prospect evaluation. Based on the latest research though, I think it’s extremely important to take point production into account when we’re assessing 17-year-olds. It’s possible that players like Rassmussen will succeed and players like Suzuki will flame out, but it’s less likely based on large samples of evidence over the past few decades. Recent history has shown us that prospects with elite point production tend to have a better chance of making it to the NHL and producing at a higher rate once they get there. Based on this information, teams can improve their chances of drafting a quality NHL player by selecting prospects at the top of this list rather than ones lower in the table.
…but context!
Remember when I said context was extremely important? This couldn’t be truer in Europe, where 17-year-olds typically get very few opportunities to succeed in professional leagues (the KHL in Russia, the SHL/Allsvenskan in Sweden, and the Finnish Liiga). Draft eligible prospects playing in these leagues will typically get fewer minutes, less PP opportunities, and lower quality linemates than they probably should based on their talent level. Because of this, the league adjustments for these players don’t do an adequate job of assessing their ability.
To help explain this dilemma, let’s look at two of my favourite Swedish prospects. After adjusting for league and age, Lias Andersson produced 0.84 Adjusted PPG in the SHL, while Jesper Boqvist produced 0.81 Adjusted PPG in the Allsvenskan, leaving them both outside of the Top 30. Let’s compare that to their production in junior (SuperElit) last year, where they absolutely tore it up. In their Draft -1 seasons, they put up 1.12 and 1.08 Adjusted PPG respectively, which would rank 8th and 10th among draft-eligible forwards. Try to keep this in mind when you’re looking at these league adjustments – it’s much more difficult for a 17-year-old prospect to succeed in a professional league than in a junior league, even after adjusting for league strength. This may sound like common sense, but it’s easy to forget about when we simply sort players by Adjusted PPG.
That was fun, let’s move onto the Top 30 blueliners.
Defensemen

Any time I talk about defensive prospects’ point production, I like to bring up this fantastic piece by Garret Hohl. The biggest takeaway from the article is that point production really matters when forecasting the probability of NHL success, even for defensemen. Hohl breaks down a great study by the aforementioned Computer Boy™, Rhys Jessop, who separated defensemen into two groups: scorers (over 0.6 adj PPG) and non-scorers (less than 0.6 adj PPG). Here’s a great visual of his findings:

This graph is immensely interesting, as it tells you two things:1. Scouts can legitimately evaluate which players have the non-scoring factors that garners success.2. Scouts are overvaluing those factors relative to scoring performance.A top-selected (picks 1-25) who was below Jessop’s arbitrary scoring line of 0.6 points per game were successful picks just as often as the above mid-selected (picks 26-50) 0.6 points per game scoring players. That’s a steep over estimation of talent.
To help make explain how we can use this knowledge to our advantage, let’s use an example from this year’s draft. I’m not suggesting that you should take Juho Korhonen (ranked outside the Top 100 by Future Considerations) over Miro Heiskanen (ranked 3rd) simply because he scored at a higher rate. Heiskanen’s ranked that high for a reason; he has many impressive traits that don’t necessarily show up in his point production (which by the way, is very impressive for a 17-year-old playing in a professional league). Scouts clearly don’t think as highly of Korhonen’s qualitative attributes, so it would be a massive reach to select him in the first couple rounds. When picking in the later rounds though (after pick 100), it’s probably a smart bet to select a player like Korhonen ahead of a defenseman with poor point production, since the research indicates he’ll have a higher chance of becoming a full-time NHLer.
One quick look at the Top 30 D in Adjusted PPG helps explain my unconditional love for Erik Brannstrom, as well as smart 2nd round bets like Connor Timmins, Markus Phillips, and Noel Hoefenmayer. On the flip side, there don’t appear to be any non-scorers with 1st round grades like Logan Stanley last year. Any team looking for a defenseman in the 1st round (ie. Toronto) appears to have a solid set of scorers to choose from, with no obvious ‘player to avoid’ that has a significantly lower likelihood of becoming an NHL player based on their lack of point production (ie. Jared Cowen, Stuart Percy, Samuel Morin, or Logan Stanley).
When it comes to the gap between “scorers” and “non-scorers” on defense, keep in mind that the 0.6 Adjusted PPG cutoff that we previously discussed is arbitrary (going from 0.61 to 0.59 Adjusted PPG doesn’t magically turn you into a poor point producer). Scoring is a continuous measure, but Jessop had to choose a cutoff to make two separate groups in his study, so he went with 0.6. Just keep in mind that all of the draft eligible defensemen in the 0.5 range this season are still pretty solid point producers even though they don’t meet Jessop’s “scorer” criteria. Also, just a quick reminder that 17-year-olds playing in professional leagues (ie. Liiga) are naturally going to have a more difficult time producing points even after adjusting for league strength, like we touched on earlier.
Concluding Thoughts
Scoring isn’t the only thing that matters in prospect evaluation, but it’s extremely important (and currently very undervalued). There tends to be a divide in hockey these days over the “analytics vs eye test debate”, but I try to avoid thinking of it in such a dichotomous fashion. At the end of the day, our goal is to make informed decisions. To accomplish this, we need to take advantage of all the information that’s available to us, which includes both the qualitative inputs from highly knowledgeable scouts (“the eye test”) and the quantitative outputs derived from statistical analysis (#analytics).
Although research has proven that scouts can identify traits that make a prospect more likely to become an NHL player, the evidence indicates that they’re often overvalued relative to point production. This presents teams with an opportunity to take advantage of this inefficiency, especially with high scoring players in European leagues (ie. Pavel Shen & Juho Korhonen). To make myself clear, I’m not suggesting that we simply rank players by Adjusted PPG and call it a day. In order to maximize drafting efficiency, we need to rely on both traditional scouting and statistical analysis. Based on the latest draft research, teams would be best served by using scouts’ knowledge to determine an approximate range of where a prospect ‘should’ be selected, then select the player in that range with the highest Adjusted PPG (or one of the highest Adjusted PPG in conjunction with elite qualitative traits such as skating or skill).
So should we use “the eye test” or “analytics” to arrive at decisions? Here’s a crazy thought: both.
Recent articles from Ian Tulloch